Commonplace
The Signal and the Noise
Nate Silver, 2012
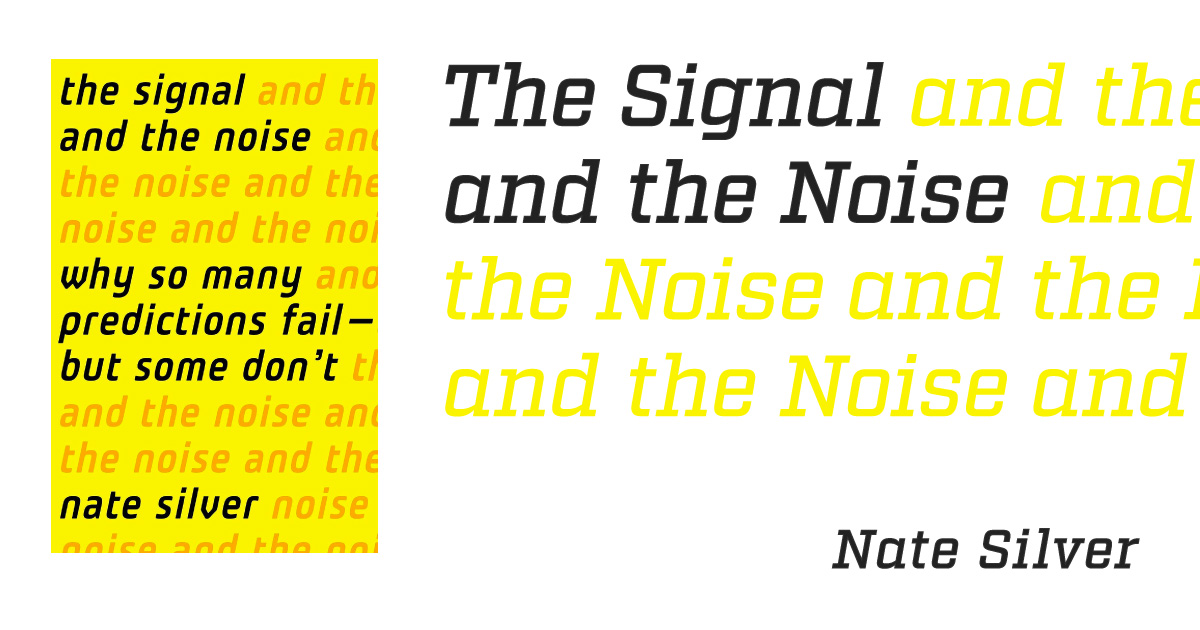
Collected excerpts, snippets, and things of interest from The Signal and the Noise by Nate Silver.
Nicholas Molina
July 20, 2023
Why I read this book
I started paying attention to Nate Silver over a decade ago and it had nothing to do with politics. I was looking for my first New York City apartment at the time and stumbled upon a neighborhood ranking model Silver built for New York magazine. I used Silver’s model to build my own, eventually landing an apartment in Bay Ridge, a neighborhood I could afford, grew to love, and wouldn’t have considered if I never read Silver’s article.
As a (now rehabilitated) political junkie, I had probably read Silver’s work before that. It’s hard to believe I missed his 2012 presidential forecast in The New York Times. More recently, like other junkies, I refreshed his 2016 election forecast at FiveThirtyEight far more times than I’d care to admit. Silver’s 2016 model faced relentless criticism for giving Trump much better odds than the competition. But Silver stood by his forecast, and eventually, Trump’s surprise win vindicated his model and cemented Silver’s place as one of the great forecasters of our time.
The power of prediction has been a revered skill throughout history. The ability to forecast the future is incredibly useful in politics, business, and everyday life (like finding an apartment). So who better to learn the powers of prediction from than the statistical genius Nate Silver?
Should you read this book?
Yes. If you have any curiosity whatsoever about how good predictions are made.
The Signal and the Noise is well written, each chapter using a different domain (sports, meteorology, economics, politics, terrorism) to explore why predictions in that domain tend to succeed or fail. Density-wise, it’s a medium read, falling somewhere between “Freakonomics” and “Thinking Fast and Slow” as far as demands on your attention. This is high praise as Silver gets us pretty deep into the statistical weeds while keeping things fun and engaging.
Silver believes strongly in Bayesian reasoning or expressing beliefs as probabilities instead of certainties and updating those beliefs when we receive new information. The world is an uncertain place and we should accept that uncertainty and think in terms of probabilities instead of absolutes. Our minds are full of biases, and humans have the unfortunate tendency to fit data to our thinking instead of our thinking to the data. If we want to make better forecasts—to get the signal from the noise—we need to be humble in the face of uncertainty, put data into a broader context, and recognize our biases so they don’t lead us astray.
Commonplace
[With the advent of the] printing press… almost overnight, the cost of producing a book decreased by about three hundred times, so a book that might have cost $20,000 in today’s dollars instead cost $70.
As was the case during the early days of the World Wide Web, however, the quality of the information was highly varied. While the printing press paid almost immediate dividends in the production of higher quality maps, the bestseller list soon came to be dominated by heretical religious texts and pseudoscientific ones.
The instinctual shortcut we take when we have “too much information” is to engage with it selectively, picking out the parts we like and ignoring the remainder…
The computer boom of the 1970s and 1980s produced a temporary decline in economic and scientific productivity. Economists termed this the productivity paradox.
The numbers have no way for speaking for themselves. We speak for them. We imbue them with meaning.
Our naive trust in models, and our failure to realize how fragile they were to our choice of assumptions, yielded disastrous results.
In 2005… John P. Ioannidis published a controversial paper titled “Why Most Published Research Findings are False.” The paper studied positive findings documented in peer-reviewed journals: descriptions of successful predictions of medical hypotheses carried out in laboratory experiments. It concluded that most of these findings were likely to fail when applied in the real world. Bayer Laboratories recently confirmed Ioanidis’s findings. They could not replicate about two-thirds of the positive findings claimed in medical journals.
The human brain is quite remarkable, it can store perhaps three terabytes of information. And yet that is only about one-millionth of the information that IBM says is now produced in the world each day.
Alvin Tofffler, writing in the book Future Shock in 1970, predicted some of the consequences of what he called “information overload.” He thought our defense mechanism would be to simplify the world in ways that confirmed our biases, even as the world itself was growing more divers and more complex.
The printing press changed the way in which we made mistakes. Routine errors of transcription became less common. But when there was a mistake, it would be reproduced many times over, as in the case of the Wicked Bible.
We can never make perfectly objective predictions. They will always be trained by our subjective point of view.
Some of the most fundamental questions that underlie the prediction problem. How can we apply our judgement to the data—without succumbing to our biases? When does market competition make forecasts better—and how can it make them worse? How do we reconcile the need to use the past as a guide with our recognition that the future may be different?
[Before the 2008 financial crisis, S&P] told investors… when it created a… collateralized debt obligation (CDO) at AAA, there was only a 0.12 percent probability—about 1 chance in 850—that it would fail to pay out over the next five years. This supposedly made it as safe as a AAA-rated corporate bond and safer than S&P now assumes U.S. Treasury bonds to be. … the actual default rates for CDOs were more than two hundred times higher than S&P predicted.
The CEO of Moody’s… explicitly told his board that ratings quality was the least important factor driving the company’s profits.
Instead their equation was simple. The rating agencies were paid by the issuer of the CDO every time they rated one: the more CDOs, the more profit. A virtually unlimited number of CDOs could be created by combining different types of mortgages—or when that got boring, combining different types of CDOs into derivatives of one another.
In what it claimed was a nod to transparency, S&P provided the issuers with copies of their ratings software. This made it easy for the issues to determine exactly how many bad mortgages they could add to the pool without seeing its rating decline.
Human beings have an extraordinary capacity to ignore risks that threaten their livelihood, as though this will make them go away.
If you make the wrong assumptions, your model may be extraordinarily wrong.
In a broader sense, the ratings agencies’ problem was being unable or uninterested in appreciating the distinction between risk and uncertainty. Risk… is something that you can put a price on… Uncertainty, on the other hand, is risk that is hard to measure.
An American home has not, historically speaking, been a lucrative investment. In fact, according to an index developed by Robert Shiller and his colleague Karl Case, the market price of an American home has barely increased at all over the long run. After adjusting for inflation, a $10,000 investment made in a home in 1896 would be worth just $10,600 in 1996.
“If you’re in a market and someone’s trying to sell you something which you don’t understand, you should think that they’re selling you a lemon” - George Akerlof
In a market plagued by asymmetries of information, the quality of goods will decrease and the market will come to be dominated by crooked sellers and gullible or desperate buyers.
When there is an excess of greed in the system, there is a bubble. When there is an excess of fear, there is a panic.
There is a common thread among these failures of prediction. In each case, as people evaluated the data, they ignored a key piece of context. … There is a technical term for this type of problem: the events these forecasters were considering were out of sample. … Forecasters often resist considering these out-of-sample problems. When we expand our sample to include events further apart from us in time and space, it often means we will encounter cases in which the relationships we are studying did not hold up as well as we are accustomed to. The model will seem less powerful. … We will be forced to acknowledge that we know less about the world than we thought we did. Our personal and professional incentives almost always discourage us from doing this.
Precise forecasts masquerade as accurate ones, and some of us get fooled and double-down our bets.
[Political experts] regardless of their occupation, experience, or subfield—had done barely any better than random chance, and they had done worse than even rudimentary statistical methods at predicting future political events. … It didn’t matter whiter the experts were making predictions about economics, domestic politics, or international affairs; their judgement was equally bad across the board.
The fox knows many little things, but the hedgehog knows one big thing
Foxes, Tetlock found, are considerably better at forecasting than hedgehogs. … [But] big, bold, hedgehog-like predictions … are more likely to get you on television.
How Foxes Think | How Hedgehogs Think |
---|---|
Multidisciplinary | Specialized |
Adaptable | Stalwart |
Self-critical | Stubborn |
Tolerant of complexity | Order-seeking |
Cautious | Confident |
Empirical | Ideological |
Foxes are better forecasters | Hedgehogs are weaker forecasters |
Hedgehogs who have lots of information construct stories—stories that are neater and tidier than the real world, with protagonists and villains, winners and losers, climaxes and denouncements…
Political news, and especially the important news that really affects the campaign, proceeds at an irregular pace. But news coverage is produced every day. Most of it is filler, packaged in the form of stories that are designed to obscure its unimportance.
Our brains, wired to detect patterns, are always looking for a signal, when we should appreciate how noisy the data is.
“When the facts change, I change my mind. What do you do, sir?” - John Maynard Keynes
Wherever there is human judgement there is the potential for bias. The way to become more objective is to recognize the influence that our assumptions play in our forecasts and to question ourselves about them.
[To think like a fox] You will need to learn how to express—and quanitfy—the uncertainty in your predictions. You will need to update your forecast as facts and circumstances change. You will need to recognize that there is wisdom in seeing the world from a different viewpoint. The more you are willing to do these things, the more capable you will be of evaluating a wide variety of information without abusing it.
[Baseball player Pedroira] doesn’t give a shit. I would come to realize that without that attitude, Pedroira might have let the scouting reports go to his head and never have made the big leagues.
Although [baseball] scouts’ judgment is sometimes flawed, they were adding plenty of value: their forecasts were about 15 percent better than the ones that relied on statistics alone.
“Is there a desire to succeed to the degree that there’s a failure mechanism kicking in? Is there a fear of failure? Is the desire to succeed significant enough to overcome the fear of failure?” - John Sanders, LA Dodgers scout on competitiveness and self-confidence, a psychological ability he believes predicts success in the major leagues
The litmus test for whether you are a competent forecaster is if more information makes your predictions better.
Our first instinct is to place information in categories… this might work well enough most of the time. But when we have rouble categorizing something, we’ll often overlook it or misjudge it.
[Don’t let] data dictate [your] decision without placing it in a larger context.
Good innovators typically think very big and they think very small. New ideas are sometimes found in the most granular details of a problem where few others bother to look. And they are sometimes found when you are doing your most abstract and philosophical thinking.
Meteorology is a field in which there has been considerable, even remarkable, progress. The power of… supercomputers is a big part of the reason why.
We may regard the present state of the universe as the effect of its past and the cause of its future. An intellect which at a certain moment would now all forces that set nature in motion, and all positions of all items of which nature is composed, if this intellect were also vast enough to submit these data to analysis, it would embrace in a single formula the movements of the greatest bodies of the universe and those of the tiniest atom; for such an intellect nothing would be uncertain and the future just like the past would be present before its eyes - Pierre-Simon Laplace
Computers aren’t good at every task we hope they might accomplish and have been far from a panacea for prediction. But computers are very good at computing: at repeating the same arithmetic tasks over and over again and doing so quickly and accurately. Tasks like chest that abide by relatively simple rules, but which are difficult computationally, are right in their wheelhouse.
Chaos theory applies to systems in which each of two properties hold:
- The systems are dynamic, meaning that the behavior of the system at one point in time influences its behavior in the future;
- And they are nonlinear, meaning they abide by exponential rather than additive relationships.
The most basic tenant of chaos theory is that a small change in initial conditions—a butterfly flapping its wings in Brazil—can produce a large and unexpected divergence in outcomes—a tornado in Texas. This does not mean that he behavior of the system is random, as the term “chaos” might imply. Nor is chaos theory some modern recitation of Murphy’s Law. It just means that certain types of systems are very hard to predict.
The problem begins when there are inaccuracies in our data [or in our assumptions]. … [Linear operations are pretty forgiving]. Exponential operations, however extract a lot more punishment… the inaccuracy quickly gets worse if the process is dynamic, meaning the outputs at one stage of the process become our inputs in the next.
According to [NWS] statistics, humans improve the accuracy of precipitation forecasts by about 25 percent over the computer guidance alone.
In contrast to most of its counterparts around the world, the Weather service is supposed t provide the model data fee of charge to anyone who wants it. Private companies like AccuWeather and the Weather Channel can then piggyback off their handiwork to develop their own products and sell them commercially.
One sign that you have made a good forecast is that you are equally at peace with however things turn out
The statistical reality of accuracy isn’t necessarily the governing paradigm when it comes to commercial weather forecasting. It’s more the perception of accuracy that adds value in the eyes of the consumer. … Most commercial weather forecasts are biased… toward forecasting more precipitation than will actually occur—what meteorologists call a “wet bias.”
One of the most important tests of a forecast is called calibration. Out of all the items you said there was a 40 precent chance of rain, how often did rain actually occur? If, over the long run, it really ddi rain about 40 percent of the time, that means you forecasts were well calibrated. If it wound up raining just 20 percent of the time instead, or 60 percent of the time, they weren’t.
“No forecast is complete without some description of… uncertainty”
When catastrophe strikes, we look for a signal in the noise—anything that might explain the chaos that we see all around us and bring order to the world again.
The official position of the USGS is [that] earthquakes cannot be predicted.
A popular misconception about earthquakes [is] that they come at regular intervals and that a region could be “due” for one if it hasn’t experienced an earthquake in some time.
In statistics, the name given to the act of mistaking noise for a signal is overfitting.
[Overfitting] is an overly specific solution to a general problem [and] it leads to worse predictions.
An overfit model… means that you’re fitting the noise in the data rather than discovering its underlying structure.
Overfitting… makes our model look better on paper but perform worse in the real world.
[Overfitting] plays into our tendency to be fooled by randomness.
Parched for prediction we will pursue it, even if it is a mirage.
A statistician drowned crossing a river that was only three feet deep on average.
Economists have for a long time been much too confident in their ability to predict the direction of the economy. … In reality, when a group of economists give you their GDP forecast, the tire 90 percent prediction interval—based on how these forecasts have actually performed and not on how accurate the economist claim them to be—span about 6.4 points of GDP [or +/- 3.2%]. When you hear the economy could grow by 2.5 percent next year, that means it could quite easily grow at a spectacular rate of 5.7 percent instead. Or it could fall by 0.7 percent—a fairly serious recession.
Economists aren’t unique… overconfident predictions has been identified in many other fields, including medical research, political science, finance, and psychology.
“Nobody has a clue. It’s ugly difficult to forecast the business cycle. Understanding an organism as complex as the economy is very hard.” - Jan Hatzius, Chief Economist at Goldman Sachs
As Hatzius sees it, economic forecasts face three challenges. First, it is very hard to determine cause and effect from economic statistics alone. Second, the economy is always changing, so explanations of economic behavior that hold in one business cycle may not apply to future ones. And third, as bad as forecasts have been, the data that economists have to work with isn’t much good either.
There have been only eleven recessions since World War II. If you have a statistical model that seeks to explain eleven outputs but has to choose from among four million inputs to do so, many of the relationships it identifies are going to be spurious. (This is a classic case of overfitting—mistaking noise for signal).
Hatzius [says the most substantive variables for economic forecasting are] growth, jobs, inflation, interest rates, wages and income, consumer confidence, industrial production, sales and consumer spending, asset prices, commodity prices, and measures of fiscal policy and government spending.
Goodhart’s law… holds that once policy makers begin to target a particular variable, it may begin to lose value as an economic indicator. … At its logical extreme, this is a bit like the observer effect: once we begin to measure something, its behavior starts to change.
A forecaster should almost never ignore data, especially when she is studying rare events like recessions or presidential elections, about which there isn’t very much data to begin with. Ignoring data is often a tip-off that the forecaster is overconfident, or is overfitting her model—that she is interested in showing off rather than trying to be accurate.
The American economy is constantly evolving… This isn’t baseball, where the game is always played by the same rules. The problem with this is that you never know when the next paradigm shift will occur.
“Why do people not give [confidence] intervals? Because they’re embarrassed” Hatzius says.
Most economic data series are subject to revision, a process that can go on for months and even years after the statistics are first published. The revisions are sometimes enormous. … Between 1965 and 2009, the government’s initial estimates of quarterly GDP were eventually revised, on average, by 1.7 points. That is the average change; the range of possible changes in each quarterly GDP is higher still, and t**he margin of error on an initial quarterly GDP estimate is plus or minus 4.3 percent. **
Meteorologists have a strong fundamental understanding of what causes tornadoes to form and what causes them to dissipate. Economics is a much softer science.
If you just look at the economy as a series of variables and equations without any underlying structure, you are almost certain to mistake noise for a signal and may delude yourself (and gullible investors) into thinking you are making good forecasts when you are not.
This kind of statement is becoming more common in the age of Big Data. Who needs theory when you have so much information? But this is categorically the wrong attitude to take toward forecasting, especially in a field like economics where the data is so noisy. Statistical inferences are much stronger when backed up by a theory or at least some deeper thinking about their root causes.
When you have your name attached to a prediction, your incentives may change. For instance, if you work for a poorly known firm, it may be quite rational for you to make some wild forecasts that will draw big attention when they happen to be right, even if they aren’t going to be right very often. Firms like Goldman Sachs, on the other hand, might be more conservative in order to stay within the consensus… [One study termed this phenomenon] “rational bias.” The less reputation you have, the less you have to lose by taking a big risk when you make a prediction. … Conversely, if you already established a good reputation, you might be reluctant to step too far out of line even when you think the data demands it.
Things like Google search traffic patterns… can serve as leading indicators for economic data series like unemployment.
“The more fundamental problem is that we have a demand for experts in our society but we don’t actually have that much of a demand for accurate forecasts.” - Robin Hanson
Danger lurks, in the economy and elsewhere, when we discourage forecasters from making a full and explicit account of the risks inherent in the world around us.
The [1976] swine flu fiasco… was a disaster on every level for President Ford… Within a couple of years, the number of Americans willing to take flu shots dwindled to only about one million…
The influenza virus is perpetuated by birds—particularly seafaring birds… they pass it along to other species, especially pigs and domesticated fowl like chickens, which live in closer proximity to humans… The perfect incubator for swine flu, then, would be a region in which each of the three conditions held: [1. A place where pigs and humans live in close proximity, 2. Near the ocean and seafaring birds, 3. In the developing world].
Extrapolation is a very basic method of prediction—usually, must too basic. It simply involves the assumption that the current trend will continue indefinitely, into the future. Some of the best-known failures of prediction have resulted from applying this principle too liberally.
Extrapolation tens to cause its greater problems in fields—including population growth and disease—where the quantity that you want to study is growing exponentially.
Precise predictions aren’t really possible to begin with when you are extrapolating on an exponential scale.
One of the most useful quantities for predicting disease spread is a variable called the basic reproduction number. Usually designated as R0, it measures the number of uninfected people that can expect to catch the disease from a single infected person… The problem is that reliable estimates of R0 can usually not be formulated until well after a disease has swept through a community.
In many cases involving predictions about human activity, the very act of prediction can alter the way that people behave. Sometimes, as in economics, these changes in behavior can affect the outcomes of the prediction itself, either nullifying it or making it more accurate.Predictions about the flu and other infectious diseases are affected by both sides of this problem.
While simplicity can be a virtue for a model, a model should at least be sophisticatedly simple.
“All models are wrong, but some models are useful.” - George E. P. Box
A model is a tool to help us understand the complexities of the universe, and never a substitute for the universe itself.
The Lakers were playing before their home crowd, which psychologists have shown provides athletes with an extra burst of testosterone when they need it most.
Successful gamblers—and successful forecasters of any kind—do not think of the future in terms of no-lose bets, unimpeachable theories, and infinitely precise measurements. These are the illusions of the sucker, the sirens of his overconfidence.
Finding patterns is easy in any kind of data-rich environment; that’s what mediocre gamblers do. The key is in determining whether the patterns represent noise or signal.
Bayes’s theorem is concerned with conditional probability. That is, it tells us the probability that a theory or hypothesis is true if some event has happened.
When our priors are strong, they can be surprisingly resilient in the face of new evidence.
Usually, however, we focus on the newest or most immediately available information, and the bigger picture gets lost.
The idea behind Bayes’s theorem, however, is not that we update our probability estimates just once. Instead, we do so continuously as new evidence presents itself to us.
There isn’t any more truth in the world than there was before the Internet or the printing press. Most of the data is just noise, as most of the universe is filled with empty space.
The idea behind frequentism is that uncertainty in a statistical problem results exclusively from collecting data among just a sample of the population rather than the whole population… essentially, the frequentist approach toward statistics seeks to wash its hands of the reason that predictions most often go wrong: human error. It views uncertainty as something intrinsic to the experiment rather than something intrinsic to our ability to understand the real world.
[Frequentist methods] discourage the researcher from considering the underlying context or plausibility of his hypothesis, something that the Bayesian method demands in the form of a prior probability.
The need for prediction arises not necessarily because the world itself is uncertain but because understating it fully is beyond our capacity.
A heuristic approach to problem solving consists of employing rules of thumb when a deterministic solution to a problem is beyond our practical capacities.
The number of possibilities in an entire chess game, played to completion, is so large that it is a significant problem even to estimate it, but some mathematicians put the number as high as 1010.
Computers struggle with abstract and open-ended problems, whereas humans understand heuristics like “control the center of the board” and “keep your pawns organized” and can devise any number of creative ways to achieve them.
In any long game of chess, it is quite likely that you and your opponent will eventually reach some positions that literally no two players in the history of humanity have encountered before.
Amateurs [chess] players, when presented with a chess problem, often frustrated themselves by looking for the prefect move, rending them incapable of making any move at all. Chess masters, by contrast are looking for a good move—and certainly if at all possible the best move in a given position—they are more forecasting how the move might favorably dispose their position than trying to enumerate every possibility.
We are not capable of making perfect decisions when presented with more information than we can process in a limited amount of time. Acknowledging those imperfections may free us to make the best decisions we can in chess and in other contexts that involve forecasting.
Elite chess players tend to be good at metacognition—thinking about the way they think—and correcting themselves if they don’t seem to be striking the right balance.
Computer chess programs often prefer short-term objectives that can be broken down and quantized and that don’t require them to evaluate the chessboard as a holistic organism.
The blind spots in our thinking are usually of our own making and they can grow worse as we age.
[Garbage in, garbage out]… if you give a computer bad data, or devise a foolish set of instructions for it to analyze, it won’t spin straw into gold.
Computers are most useful to forecasters… in fields like weather forecasting and chess where the system abides by relatively simple and well-understood laws, but where the equations that govern the system must be solved many times over in order to produce a good forecast. They seem to have helped very little in fields like economics or earthquake forecasting where our understanding of root causes is blurrier and the data noisier.
Whatever biases and blind spots the forecaster has are sure to be replicated in his computer program.
The psychological factors in poker come mostly in the form of self-discipline… poker is an incredibly mathematical game that depends on making probabilistic judgements amid uncertainty, the same skills that are important in any type of prediction.
A player is blessed with a pair only rarely in [Texas] hold’em… the chance of staring out with a pair of nines or better is only about 3 percent.
While television coverage has been a great boon to poker, it leaves many casual players with misleading impressions about the right way to play it, focusing too much on the results and not enough on the correct decision-making process.
“It’s important in most areas of life to come up with a probability instead of a yes or no” - Tom Dwan
In 2004 and 2005, [Nate Silver] made an income from poker in the six figures, with [his] cumulative profits from the game peaking at about $400,000 overall.
The Pareto principle or 80-20 rule (as in: 80 percent of your profits come from 20 percent of your customers). As I apply it here, it posits that getting a few basic things right can go a long way. In poker, for instance, simply learning to fold your worst hands, bet your best ones, and make some effort to consider what your opponent holds will substantially mitigate your losses.
[The Pareto principle] also holds in many other disciplines in which prediction is vital. The first 20 percent often begins with having the right data, the right technology, and the right incentives. You need to have some information—more of it rather than less, ideally—and you need to make sure that it is quality-controlled. You need to have some familiarity with the tools of your trade—having top-shelf technology is nice, but it’s more important that you know how to use what you have. You need to care about accuracy—about getting at the objective truth—rather than about making the most pleasing or convenient prediction, or the one that might get you on television.
Sometimes… it is not so much how good your predictions are in an absolute sense that matters but how good they are relative to the competition.
In a field that is highly competitive, it is only through… painstaking effort around the margin that you can make any money… I’ve tried to avoid these sorts of areas.
It is often possible to make a profit by being pretty good a prediction in fields where the competition succumbs to poor incentives, bad habits or blind adherence to tradition… it is much harder to be very good in fields where everyone else is getting the basics right…
If you’re approaching prediction as more of a business proposition, you’re usually better off finding someplace where you can be the big fish in a small pond.
[In poker] you see the statistical echo of the 80/20 rule: there’s a much larger difference between the very worst players and the average ones than between the average ones and the best.
If you can’t spot one or two bad players in the game, you should’t be playing in it.
[Once Party Poker shut Americans out] the fragile ecology of the poker economy was turned upside down—without those weaker players to prop the game up, the water level had risen, and some of the sharks had turned into suckers.
Just as we perceive more signal than there really is when we make predictions, we also tend to attribute more skill than is warranted to successful predictions when we assess them later.
Focus more on process than results.
To the extent that markets are reflections of our collective judgement, they are fallible too.
Simply taking an average of everyone’s forecast rather than relying on just one has been found to reduce forecast error, often by about 15 or 20 percent. But first before you start averaging everything together, you should understand three things. First, while the aggregate forecast will essentially always be better than they typical individual’s forecast, that does’t necessarily mean it will be good… Second, the most robust evidence indicates that this wisdom-of-crows principle holds when forecasts are made independently before being averaged together… Third, although the aggregate forecast is better than the typical individual’s forecast, it does not necessarily hold that it is better than the best individual’s forecast.
When this property has been studied over the long run, however, the aggregate forecast has often beaten even the very best individual forecast… Reducing error by 15 or 20 percent by combining forecasts may not sound that impressive, but its awfully hard to beat in a competitive market.
Very often, we fail to appreciate the limitations imposed by small sample sizes and mistake luck for skill when we look at how well someone’s predictions have done.
There was no consistency in how well a [mutual] fund did, even over five-year increments. Other studies have identified very modest correlations in mutual fund performance from year to year, but it’s so hard to tell them apart that you’re best off selecting the ones with the cheapest fees—or eschewing them entirely and investing in the market yourself.
Members of Congress, who often gain access to insider information about a company while they are lobbied and who also have some ability to influence the fate of companies through legislation, return a profit on their investments that beats market averages by 5 to 10 percent per year.
Stock market data ultimately describes not some natural phenomenon but the collective actions of human beings. If you do detect a pattern, particularly an obvious-seeming one, the odds are that other investors have found it as well, and the signal will begin to cancel out or even reverse itself.
Of the eight times in which the S&P 500 increased in value by twice its long-term average over a five-year period, five cases were followed by a severe and notorious crash, such as the Great Depression, the dot-com bust, or [Black Monday 1987].
When the P/E ratio [of all the companies in the S&P 500] is 10, meaning that stocks are cheap compared with their earnings, they have historically produced a real return of about 9 percent per year… and when they are very high, above about 30—as they were in 1929 or 2000—the expected return has been negative.
There is very little that is truly certain about the stock market.
Reliable stock market data only goes back 120 years or so—not all that much data if you really want to know about the long run. Statistical tests suggest that he true long-run return—what we might expect over the next 120 years—could be anywhere from 3 percent to 10 percent instead of 7 percent. The answer to what economists call the “equity premium puzzle”—why stocks have returned so much more money than bonds in a way that is disproportionate to the risks they entail—may simply be that the returns stocks achieved in the twentieth century were anomalous, and the true long-run return isn to as high as 7 percent.
The answer to why bubbles form," Blodget told me, “is that it’s in everybody’s interest to keep markets going up.” … So long as most traders are judged on the basis of short-term performance, bubbles involving large deviations of stock prices from their long-term values are possible—and perhaps even inevitable.
The heuristic of “follow the crowd, especially when you don’t know any better” usually works pretty well.
Perhaps the central finding of behavioral economics is that most of us are overconfident when we make predictions.
There is reasonably strong evidence for what Thaler calls No Fee Lunch—it is difficult (although not literally impossible) for any investor to beat the market over the long-term.
There is a kind of symbiosis between the irrational traders and the skilled ones—just as, in a poker game, good players need some fish at the table to make the game profitable to play in. In the financial literature, these irrational traders are known as “noise traders.”
Any investor can do as well as the average investor with almost no effort. All he needs to do is buy and index fund that tracks the average of the S&P 500.
I pay quite a bit of attention to what the consensus view is… the further I move away from that consensus, the stronger my evidence has to be before I come to the view that I have things right and everyone else has it wrong.
We’ll never detect a bubble if we start from the presumption that markets are infallible and the price is always right. Markets cover up some of our warts and balance out some of our flaws. And they certainly aren’t easy to out predict. But sometimes the price is wrong.
A failure to think carefully about causality will lead us up blind alleys.
Water vapor, not CO2, is the larges contributor to the greenhouse effect… [But] as CO2 and other long-lived greenhouse gases increase in concentration and warm the atmosphere, the amount of water vapor will increase as well, multiplying the effects of CO2 and enhancing warming.
Scientists require a higher burden of proof before they are willing to conclude a hypothesis is incontrovertible. The greenhouse hypothesis has met this standard.
Skepticism… needs to weigh the strength of new evidence against the overall strength of the theory, rather than rummaging through fact and theory alike for argumentative and ideological convenience.
Complexity, uncertainty, and the value (or lack thereof) of consensus views are core themes of this book.
In formal usage, consensus is not synonymous with unanimity—nor with having achieved a simple majority. Instead, consensus connotes broad agreement after a process of deliberation.
[Group deliberation] introduces the possibility of groupthink and herding. Some members of a group may be more influential because of their charisma or status and not necessarily because they have the better idea. Empirical studies of consensus-driven predictions have found mixed results, in contrast to a process wherein individual members of a group submit independent forecasts and those are averaged or aggregated together, which can almost always be counted on to improve predictive accuracy.
“Different models have different coding errors. You cannot assume that a model with millions of lines of code, literally millions of instructions, that there isn’t a mistake in there.”
The improvements in weather forecasts are a result of two features of their discipline. First meteorologists get a lot of feedback—weather predictions play out daily, a reality check that helps keep them well-calibrated. This advantage is not available to climate forecasters and is one of the best reasons to be skeptical about their predictions, since they are made at scales that stretch out to as many as eighty one hundred years in advance.
I would urge caution against reducing the forecasting process to a series of bumper-sticker slogans. Heuristics like Occam’s razor… sound sexy but they are hard to apply. We have seen cases… where the assumptions of a model are simple and elegant—but where the are much too naïve to provide for very skillful forecasts. We have also seen cases… where unbelievably convoluted forecasting schemes that look great in the software package fail miserably in practice.
The sun gives off slightly more and slightly less radiation over cycles that last for about eleven years on average. (This is often measured through sunspots…)
There are periodic interruptions from volcanoes, which blast sulfur—a gas that has an anti-greenhouse effect and tends to cool the planet—into the atmosphere. The eruption of Mount Pinatubo in 1991 reduced global temperatures by about 0.2°C for a period of two years, equivalent to a decade’s worth of greenhouse warming.
One of the most tangible contributions of climate models, in fact, is that they find it impossible to replicate the current climate unless they account for the increased atmospheric concentrations of CO2 and other greenhouse gases.
When a prediction about a complex phenomenon is expressed with a great deal of confidence, it may be a sign that the forecaster has not through through the problem carefully, has overfit his statistical model, or is more interested in making a name for himself than in getting at the truth.
Uncertainty is an essential and nonnegotiable part of a forecast.
When we advance more confident claims and they fail to come to fruition, this constitutes a much more powerful evidence against our hypothesis. We can’t really blame anyone for losing faith in our forecasts when this occurs, they are making the correct inference under Bayesian logic.
In a society accustomed to overconfident forecasters who mistake the confidence they express in a forecast for its veracity, expressions of uncertainty are not seen as a winning strategy by either side [of a debate].
The dysfunctional state of the American political system is the best reason to be pessimistic about our country’s future. Our scientific and technological prowess is the best reason to be optimistic.
Worse than being unprepared, [at Pearl Harbor] we had mistaken our ignorance for knowledge and made ourselves more vulnerable as a result.
It is much easier after the event to sort the relevant from the irrelevant signals. After the event, of course, a signal is always crystal clear; we can now see what disaster it was signaling, since the disaster has occurred. But before the event it is obscure and pregnant with conflicting meanings. It comes to the observer embedded in an atmosphere of “noise,” i.e., in the company of all sorts of information that is useless and irrelevant for predicting the particular disaster.—Roberta Wohlstetter
There is a tendency in our planning to confuse the unfamiliar with the improbable. The contingency we have not considered seriously looks strange; what looks strange is though improbable; what is improbable need not be considered seriously. —Thomas Schelling
There are known knowns; there are things we know we know. We also know there are known unknowns; that is to say we know there are some things we do not know. But there are also unknown unknowns—there are things we do not know we don’t know.—Donald Rumsfeld
Anytime you are able to enumerate a dangerous or unpredictable element, you are expressing a known unknown.
The problem comes when, out of frustration that our knowledge of the world is imperfect, we fail to make a forecast at all. An unknown unknown is a contingency that we have not even considered. We have some kind of mental block against it, or our experience is inadequate to imagine it; it’s as though it doesn’t even exist.
Daniel Kahneman calls [this] the availability heuristic—we tend to overrate the likelihood of events that are nearer to us in time and space and under predict the ones that aren’t…
This type of patter—a very small number of cases causing a very large proportion of the total impact—is characteristic of a power-law distribution, the type of distribution that earthquakes obey. Clausset’s insight was that terror attacks abide by a power-law distribution as well.
Power laws have some important properties when it comes to making predictions about the scale of future risks. In particular, they imply that disasters much worse that what society has experienced in the recent past are entirely possible, if infrequent.
The September 11 attacks alone killed more people… than all other attacks in NATO countries over the thirty-year period between 1979 and 2009 combined… So if Levi is right that the odds of these attacks are extremely low, they represent the bulk of the hazard… When it comes to terrorism, we need to think big, about the probability for very large magnitude events and how we might reduce it, even at the margin. Signals that point toward such large attacks should therefore receive a much higher strategic priority.
Although Israel is targeted by terrorists much more frequently than the United States, Israelis do not live in fear of terrorism. A 2012 survey of Israeli Jews found that only 16 percent described terrorism as their greatest fear…
We just aren’t that good at prediction, and we need to hedge our bets rather than believe solely in one hypothesis…
Where our enemies will strike is predictable: its where we least expect them to.
Like a baseball umpire, an intelligence analyst risks being blamed when something goes wrong but receives little notice when she does her job well.
There is no reason to conclude that the affairs of men are becoming more predictable. … The volume of information is increasing exponentially. But relatively little of this information is useful—the signal-to-noise ratio may be waning. We need better ways of distinguishing the two.
**Slow down, and consider the imperfections in your thinking. Over time, you should find that this makes your decision making better. **
What isn’t acceptable under Bayes’s theorem is to pretend that you don’t have any prior beliefs. You should work to reduce your biases, but to say you have none is a sign that you have many.
Most of the time, we do not appreciate how noisy the data is, and so our bias is to place too much weight on the newest data point.
A sign that you’re spending too much time in model land is if you start to use the word “prediction” to refer to how your model fits past data. As I describe in chapter 5, it is very easy to overfit a model, thinking you have captured the signal when you’re just describing the noise. Sticking to the simple, commonsense definition of a prediction as something that applies strictly to a future event may reduce the risk of these errors.
**Distinguishing the signal from the noise requires both scientific knowledge and self-knowledge, the serenity to accept the things we cannot predict, the courage to predict the things we can, and the wisdom to know the difference. **